In “Potential Changes in Algorithmic Evaluation Systems: A Look into Quality Rater Research,” the article explores the potential for major changes in algorithmic evaluation processes used by search engines. It highlights recent research by Bing and other players in the field, suggesting that significant shake-ups could be on the horizon. These changes have the potential to impact both quality raters and the frequency of algorithmic updates. The article also dives into the importance of evaluation in search engine results and the role of human-in-the-loop feedback. It discusses the different types of feedback, including implicit and explicit evaluation, and the ways in which data labels are acquired. Overall, the article provides an insightful look into the evolving landscape of algorithmic evaluation systems.
The importance of evaluation
Evaluation plays a crucial role in search engines and their ability to provide relevant and helpful search results to users. The concept of relevance is inherently subjective and constantly changing, which means that search engines need to continuously evaluate their search result sets and experimental designs to ensure they align with the evolving needs and preferences of users.
Temporal query intent shifts further complicate the evaluation process. Search queries can undergo predictable, temporal shifts in intent based on factors like seasonal events or local happenings. For example, queries related to Black Friday may shift from being informational to commercially focused. These shifts require search engines to adapt their result pages to meet the changing intent of users.
To evaluate proposed changes in search result rankings or experimental designs, search engines need to determine if the proposed changes are truly better and more precise in meeting users’ information needs compared to the current results. Evaluation serves as a critical stage in the evolution of search results, providing confidence in proposed changes and generating data for further adjustments and algorithmic tuning, if necessary.
Human-in-the-loop (HITL) evaluation involves the active participation of humans in providing feedback before roll-outs to production environments. This feedback can be obtained through various methods, such as implicit and explicit evaluation feedback.
Human-in-the-loop (HITL)
In HITL evaluation, humans play a crucial role in providing feedback on the relevance of search results to queries. This feedback is obtained through explicit or implicit means.
Data labels and labeling are key components of HITL evaluation. Data labels are assigned to items to transform them into measurable forms. In the context of search engines, data labels are created when users engage with search results, such as marking an email as spam or indicating their preference for a film on a streaming platform like Netflix. These data labels help search engines understand user preferences and train their algorithms to deliver more relevant results.
Implicit feedback, on the other hand, is obtained from user behavior without their active awareness. This includes analyzing click data, user scrolling, dwell time, and result skipping. Implicit feedback provides insights into how users interact with search results and can inform the development of ranking algorithms.
Explicit feedback involves actively collecting feedback from participants who are aware of providing feedback. This feedback is often used to tune algorithms or evaluate experimental designs. There are several formats of explicit feedback, including:
-
Real users in feedback sessions with user feedback teams: Search engine user research teams collaborate with real users in feedback sessions to gather relevance data labels for queries and their intents. While this format provides near-gold standard relevance labels, it is not scalable due to its time-consuming nature and limited number of participants.
-
True subject matter experts / topic experts / professional annotators: Relevance assessors who are experts in a particular subject or topic provide relevance labels for query mappings. This type of labeling is also considered near-gold standard, but it suffers from the same scalability challenges as user feedback sessions.
-
Search engines simply ask real users whether something is relevant or helpful: Search engine users are explicitly asked whether a search result is relevant or helpful. This explicit binary feedback, such as thumbs-up or thumbs-down responses, provides valuable insights into the relevance of search results.
-
Crowd-sourced human quality raters: Crowd-sourced human quality raters, hired through external contractors and trained by search engines, provide explicit feedback in the form of synthetic relevance labels. These raters compare proposed changes in search result rankings or experimental designs to existing systems or other proposed changes, helping search engines evaluate the effectiveness of these changes.
Crowd-sourced human quality raters are a major source of explicit feedback and are employed by search engines like Google and Bing. These raters play a crucial role in evaluating search results and informing algorithmic updates. However, the scalability of this approach is limited, and the feedback provided may not always reflect the preferences and needs of the wider search population.
Algorithmic evaluation systems and their potential changes
Algorithmic evaluation systems are constantly evolving to improve precision and relevance in search results. Search engines, such as Bing, have been conducting groundbreaking research and implementing changes based on their findings. This research, coupled with the increase in closely related information retrieval research by others, indicates potential major changes in algorithmic evaluation systems.
These potential changes can have far-reaching consequences for both human quality raters and the frequency of algorithmic updates. The role of human quality raters may evolve, and the frequency of algorithmic updates may increase as search engines strive to improve the accuracy and relevance of search results.
Bing’s research and implementation efforts have focused on areas such as large-scale crowd labeling and the use of Rank-Biased Precision (RBP) scores for evaluation. These efforts highlight the ongoing use of metric ranges based on human judge-submitted relevance evaluations in a production environment.
Closely related information retrieval research by other industry players further supports the notion of potential changes in algorithmic evaluation systems. As search engines continue to explore new approaches and techniques, the role of human quality raters and the algorithmic updates they inform may undergo significant transformations.
The future of algorithmic evaluation systems
The future of algorithmic evaluation systems promises advancements in precision, relevance, and adaptation to user preferences. Machine learning plays a crucial role in these advancements, as search engines leverage large amounts of training data from explicit feedback and implicit user behavior to improve their algorithms.
Improving precision and relevance entails refining the algorithms used to determine search result rankings. By analyzing explicit feedback from crowd-sourced human quality raters, search engines can fine-tune their algorithms to deliver more accurate and relevant results.
Adapting to user preferences involves understanding and incorporating the evolving needs and preferences of search engine users. As user behavior shifts and new queries emerge, search engines must continuously adapt their algorithms to deliver the most meaningful results. Machine learning models that capture user preferences and intent play a vital role in this adaptation process.
The role of machine learning becomes central in algorithmic evaluation systems. These systems leverage machine learning models to detect patterns in user behavior and relevance feedback, enabling search engines to provide more personalized and contextually relevant search results.
Addressing bias and noise is another important consideration in the future of algorithmic evaluation systems. By improving the quality and scalability of data labeling processes and optimizing algorithms to reduce bias, search engines can enhance the fairness and accuracy of their search results.
Conclusion
Evaluation is a critical component of search engines and their ability to provide relevant and helpful search results. Human-in-the-loop evaluation, through explicit and implicit feedback, allows search engines to fine-tune their algorithms, evaluate proposed changes, and ensure the accuracy and relevance of search results.
Algorithmic evaluation systems are continuously evolving, with major changes on the horizon. Bing’s research and implementation efforts, as well as the increase in closely related information retrieval research, indicate potential transformations in these systems. These changes can have far-reaching consequences for human quality raters and the frequency of algorithmic updates.
The future of algorithmic evaluation systems holds promise in terms of improving precision, adapting to user preferences, leveraging machine learning, and addressing bias and noise. By continuously refining their algorithms and incorporating user feedback, search engines can deliver more accurate, relevant, and personalized search results to users.
Please rate this post
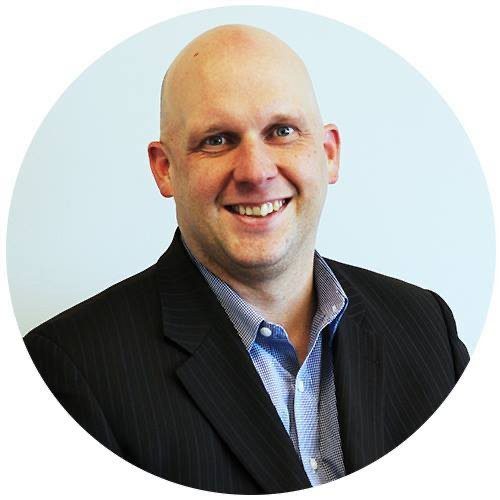
With over 20 years of experience in the car business, I’ve navigated the evolution of the industry from traditional sales to the dynamic digital age. My journey through various roles in both sales and management has endowed me with a unique perspective on the challenges and opportunities in automotive sales today.
As the founder of Shawn Ryder Digital, I combine my extensive background in technology with my deep understanding of the automotive industry. This synergy allows me to craft digital marketing strategies that are not just effective but tailored to the specific needs of each dealership. My commitment is to drive your sales, enhance your brand awareness, and ensure your dealership thrives in the digital landscape.
Here at Shawn Ryder Digital, we’re not just about providing services; we’re about building partnerships. As I often say, “In the fast-paced world of digital marketing, staying ahead isn’t just an option; it’s a necessity.”
Together, let’s embrace the challenges of the digital age and turn them into opportunities for growth and success. Join me in redefining the future of automotive digital marketing. Let’s accelerate your dealership’s journey to the top.